Knowledge Graphs, Vector Embeddings, & Semantic Search with ScholarAI
Explore how ScholarAI enhances ChatGPT-4 with knowledge graphs, vector embeddings and semantic search for accurate AI responses.
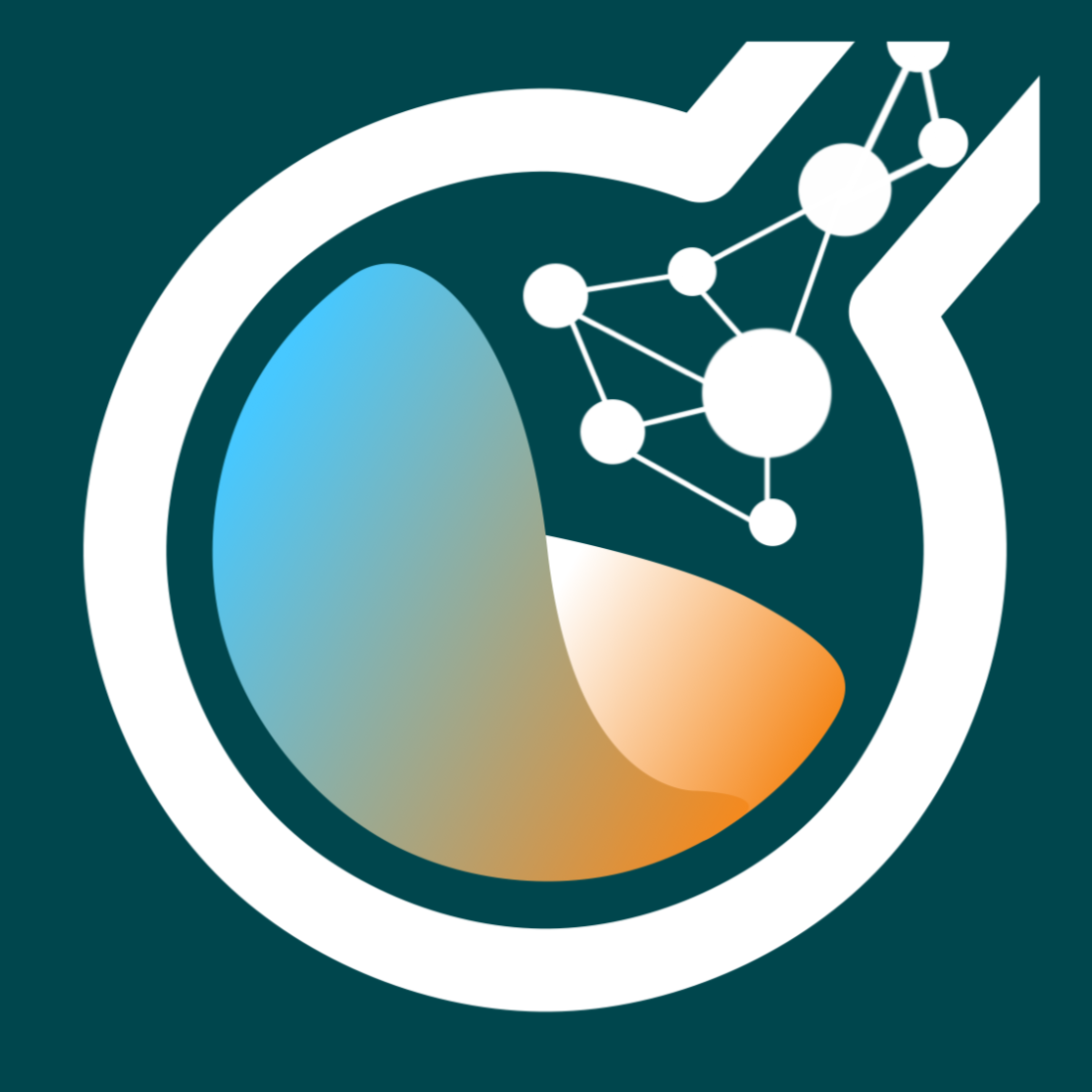
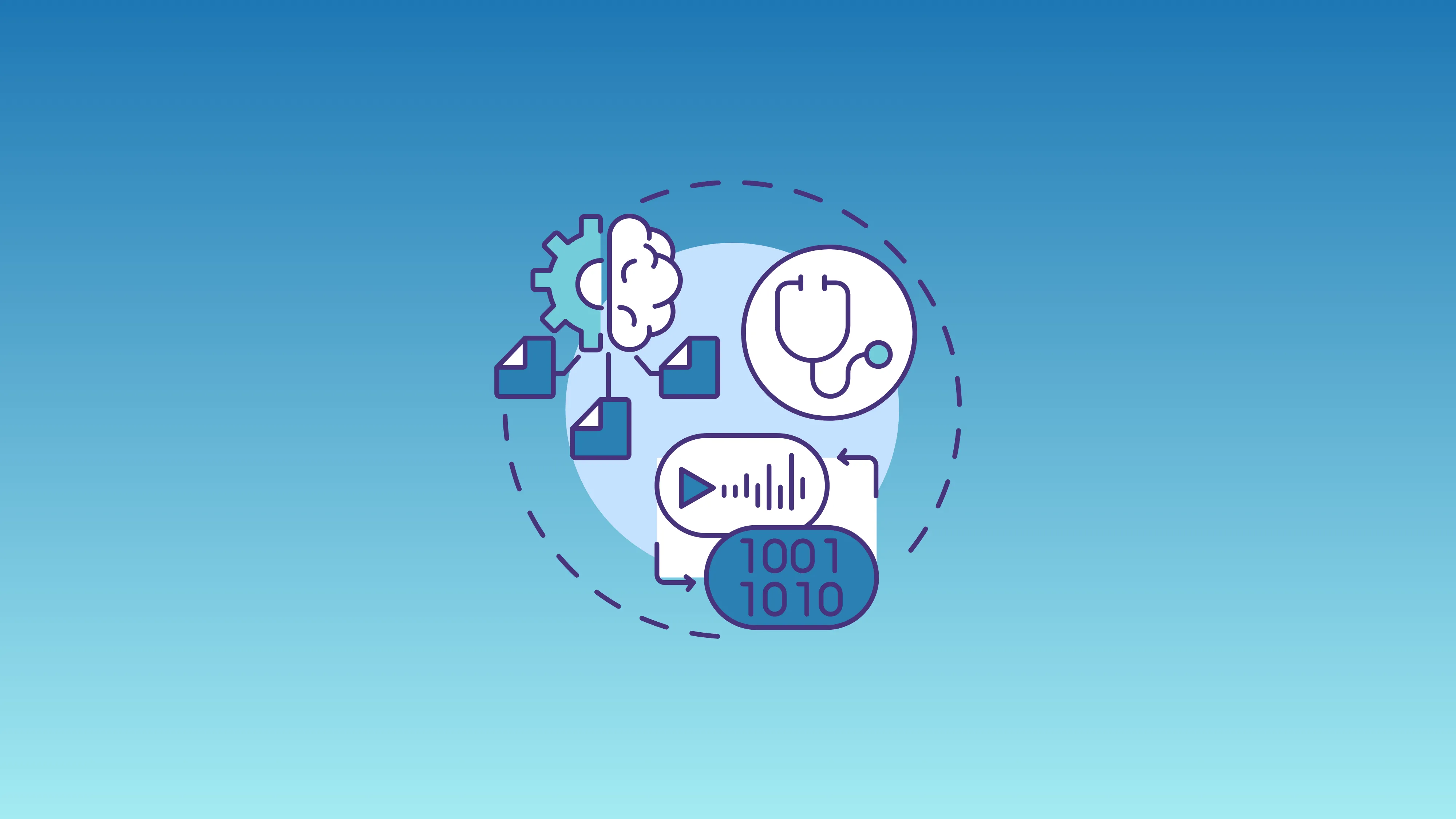
The journey towards creating AI that can accurately interpret vast datasets is a critical challenge in the development of trustworthy artificial intelligence. ScholarAI stands at the forefront of this mission, integrating with ChatGPT to significantly enhance the precision, context and trustworthiness of AI responses. This blog dives into some of the advanced methodologies at the core of ScholarAI, including our use of knowledge graphs, vector embeddings, and semantic search integrated within our tool. By leveraging these frameworks, we're building AI that goes beyond mere understanding to provide detailed, source-backed answers.
Keep reading to see how ScholarAI connects large language models (LLMs), like GPT-4 powering ChatGPT to the most important information for your prompts.
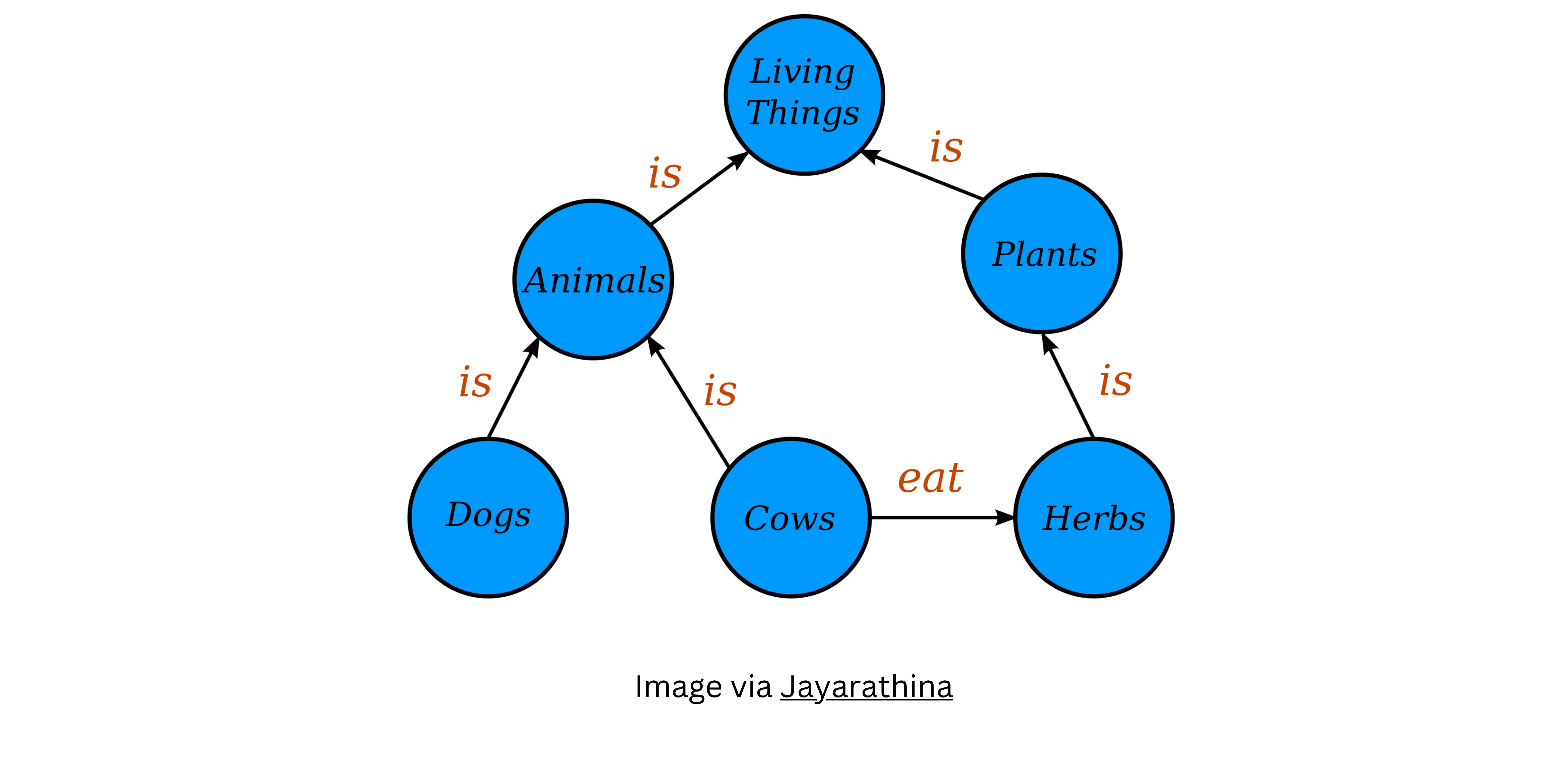
What are knowledge graphs?
Knowledge graphs are mathematical representations of entities (also known as nodes), with specific attributes, connected by context-specific relationships (also known as edges). Knowledge graphs enable information (e.g., a scientific article, or the information contained within a scientific article) to be represented with structure that can be parsed and searched by computer programs like AI.
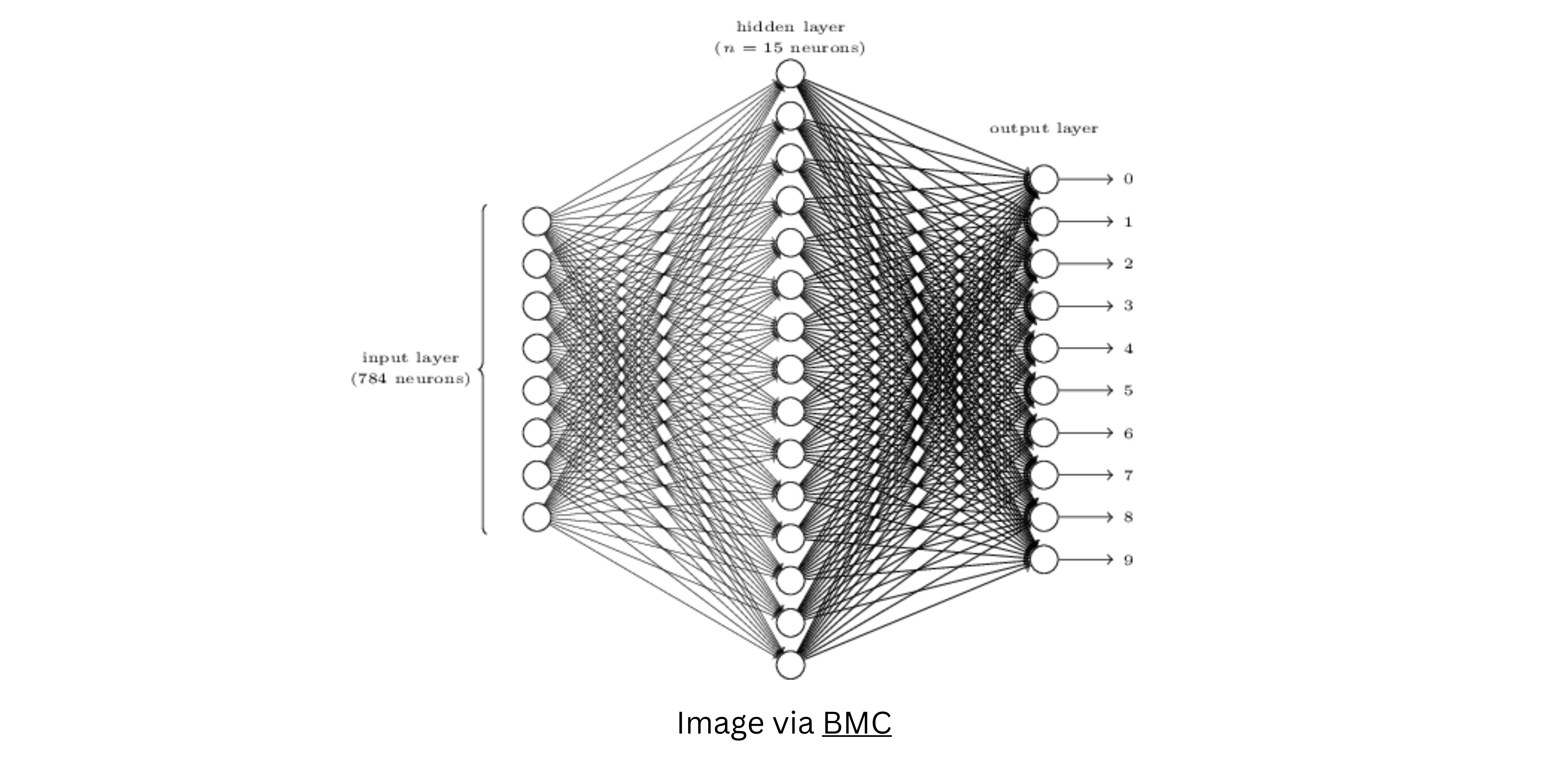
What are vector embeddings?
Vector embeddings are the numerical representation of words and phrases in high-dimensional space. In vector embeddings, words are transformed into a vector series of numbers. These vectors capture words, phrases, and semantic information, which enables the mathematical calculation of how similar information is among vectors. When properly connected, AI systems (like GPT-4 powered by ChatGPT) can use vector embeddings of text (including entire documents) to find information and return highly accurate results to user prompts.
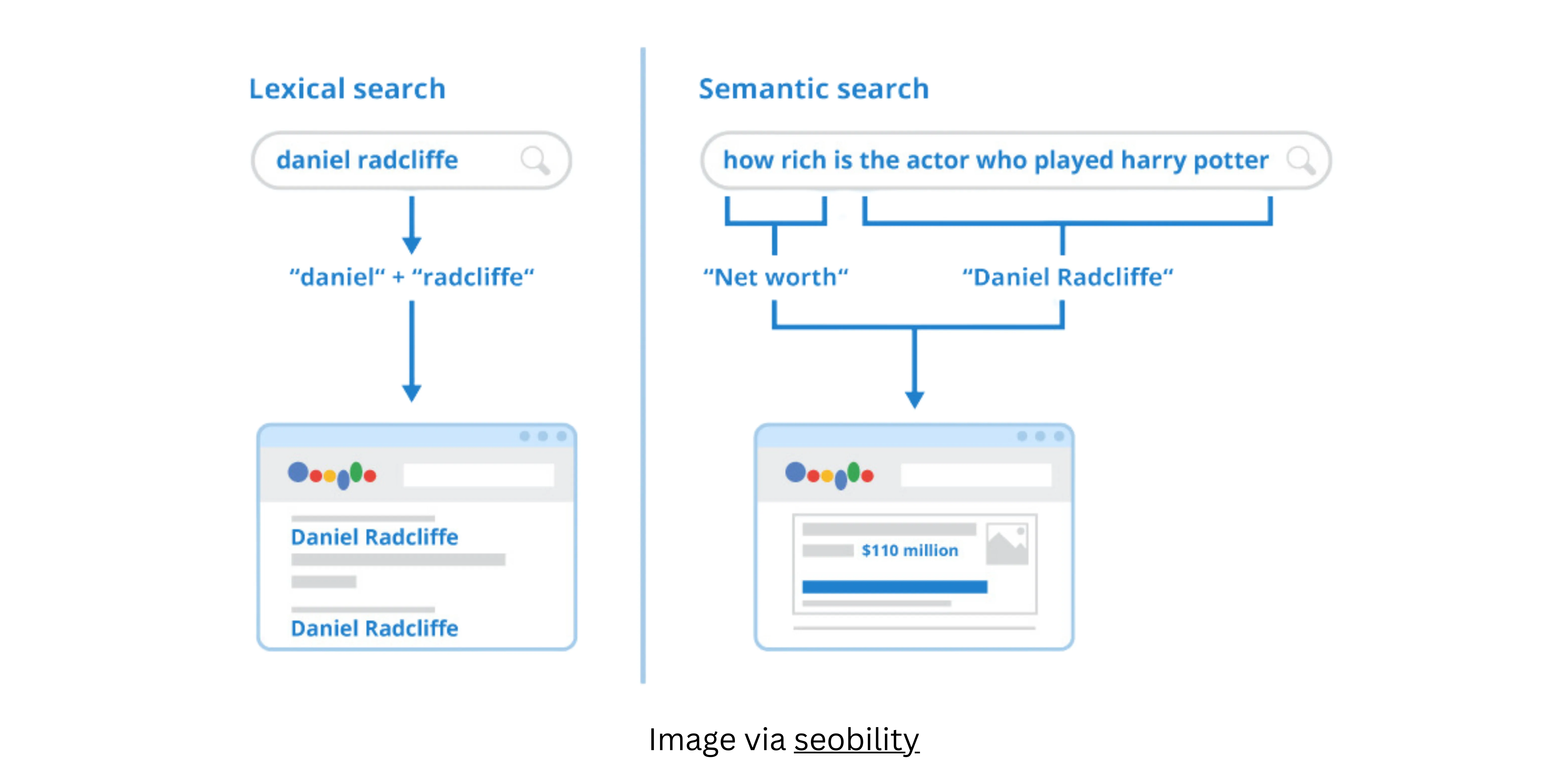
What are semantic searches?
Semantic searches are searches that consider the context and intent of search queries to return results for given prompts. Semantic search is generally considered an evolution of traditional keyword matching-based search. The increased understanding enabled by semantic searches achieves greater accuracy than other search types. Natural language processing has given rise to increased semantic search functions and contextual meaning, making this process better. Advanced, thoughtful semantic searching can enable AI to find information, synthesize it, and generate novel insights.
Where does ScholarAI come in?
Since inception, ScholarAI has connected LLMs, like GPT-4 powering ChatGPT, to knowledge graphs and vector embeddings to return AI-generated responses (e.g., paper summaries) and hyperlinked source material (e.g., papers matching user prompts). In this way, ScholarAI provides a “map” to LLMs, helping them navigate these complex spaces more accurately and with increased context specificity. As we continue to improve our system, we are engineering new methods to further improve semantic search so that large amounts of information (for example thousands of academic papers on a given topic) can be passed to LLMs to generate thorough and accurate information synthesis.
Conclusion
Our integration with ChatGPT-4 represents a significant advancement in AI's ability to deliver accurate and contextually relevant information. Through the strategic use of knowledge graphs, vector embeddings, and semantic search, we're ensuring that users receive responses that are not only precise but also deeply informed. As we continue to refine these tools, the path to a partnership with trustworthy AI in research and analysis becomes ever clearer.
Interested in adding a trustworthy AI tool to your toolbelt? Join our journey toward reliable and accurate AI - sign up for ScholarAI here.