A ChatGPT Plugin: ScholarAI's Role in Smarter Research
From the roots of LLMs to ScholarAI's integration with ChatGPT— Learn about our history and how to transform your research workflow with ScholarAI.
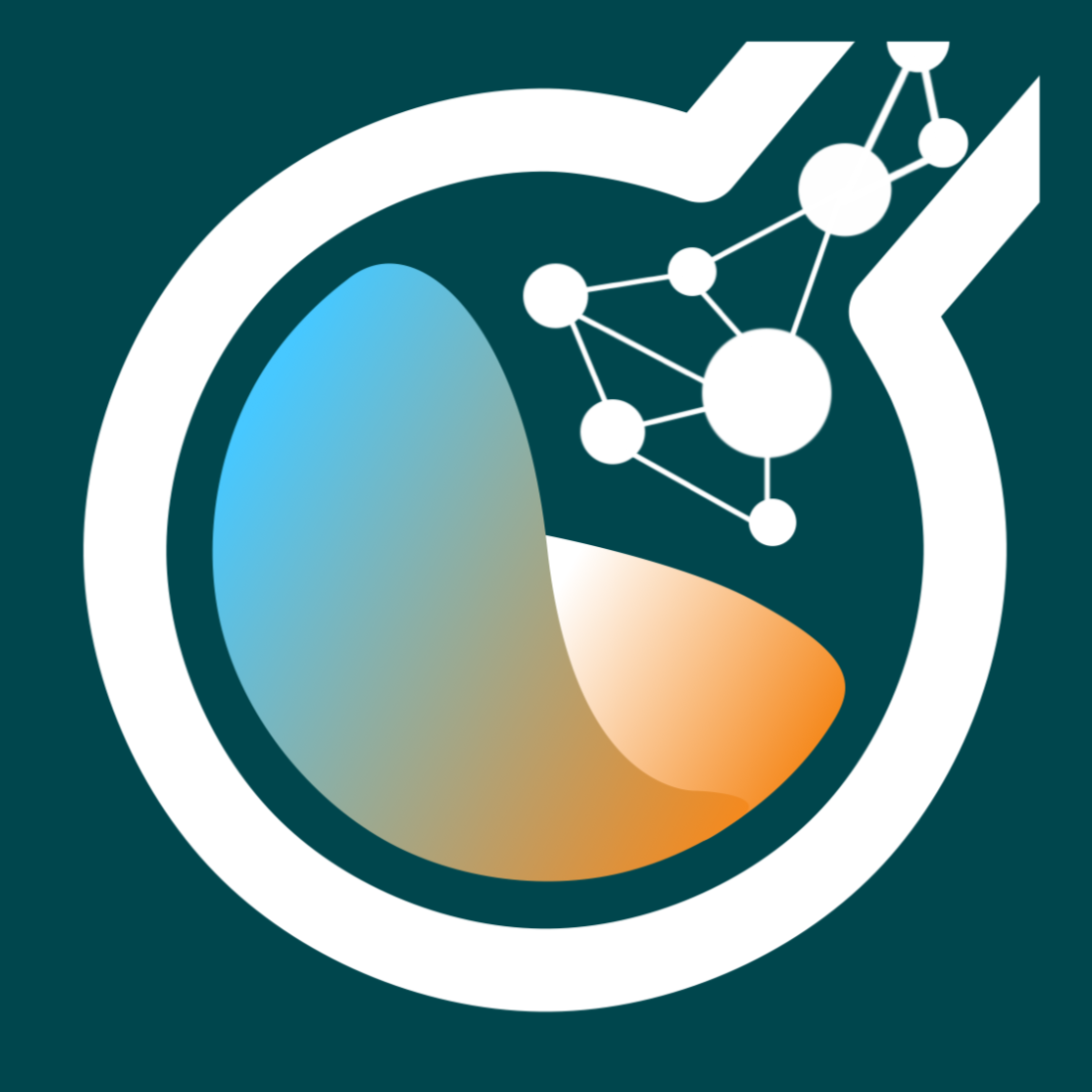
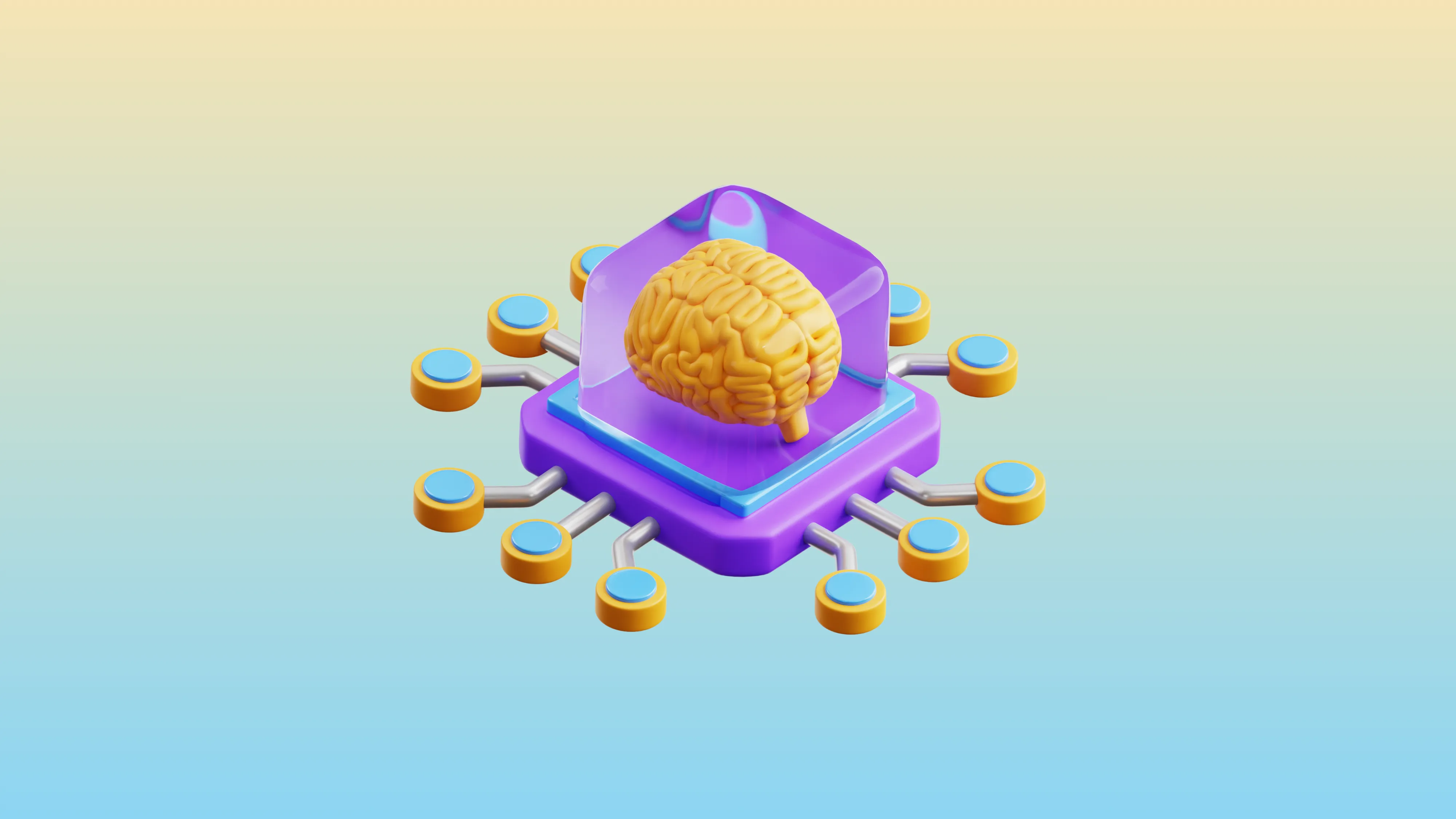
In a digital era where information is abundant yet not always reliable, ScholarAI emerges as a beacon of trust and efficiency for researchers in science, medicine, business, and law. Our ChatGPT plugin stands as a testament to this, providing an AI-driven research assistant that navigates over 200 million peer-reviewed articles, and now integrated with the Zotero citation manager. This blog highlights the evolution of large language models (LLMs) like GPT and explores how ScholarAI leverages these advancements to offer a more seamless and intuitive research experience.
What are Large Language Models (LLMs)?
Large language models (LLMs) are a type of artificial intelligence (AI) that can generate text, translate languages, write different kinds of creative content, and answer specific and open-ended questions[1]. LLMs are trained on massive amounts of text data (usually publicly-available internet data), and can “learn” to perform a wide range of tasks.
LLMs use statistical models to analyze data, learning the patterns and connections between words and phrases[2]. Many LLMs are based on a transformer architecture described most notably in a seminar paper called “Attention Is All You Need” which can be found here.
LLMs can be trained to find previously unknown patterns in data. Unsupervised and semi-supervised training eliminates the need for extensive data labeling, which is one of the biggest challenges in building high-accurate AI models. Advances in these techniques have quicken the pace of innovation for LLMs, and have also contributed to the advancement of other forms of AI generally. Extensive reviews about unsupervised and semi-supervised learning exists, and for those interested, we recommend beginning your reading here and here.
LLMs in Research and Medicine
As described by Qureshi et al., LLMs have demonstrated limited usefulness in research and medicine thus far and remain incomplete, in their current forms, as clinical tools. As we and others have previously described, a major hurdle for LLMs remains their trustworthiness (i.e., their current affinity for hallucinations). ScholarAI is dedicated to being at the forefront of building trustworthy AI systems.
For those interested, a review paper titled: The Utility of ChatGPT as an Example of Large Language Models in Healthcare Education, Research and Practice: Systematic Review on the Future Perspectives and Potential Limitations, by Malik Sallam is helpful in providing additional context for some of the challenges AI is facing in technical fields.
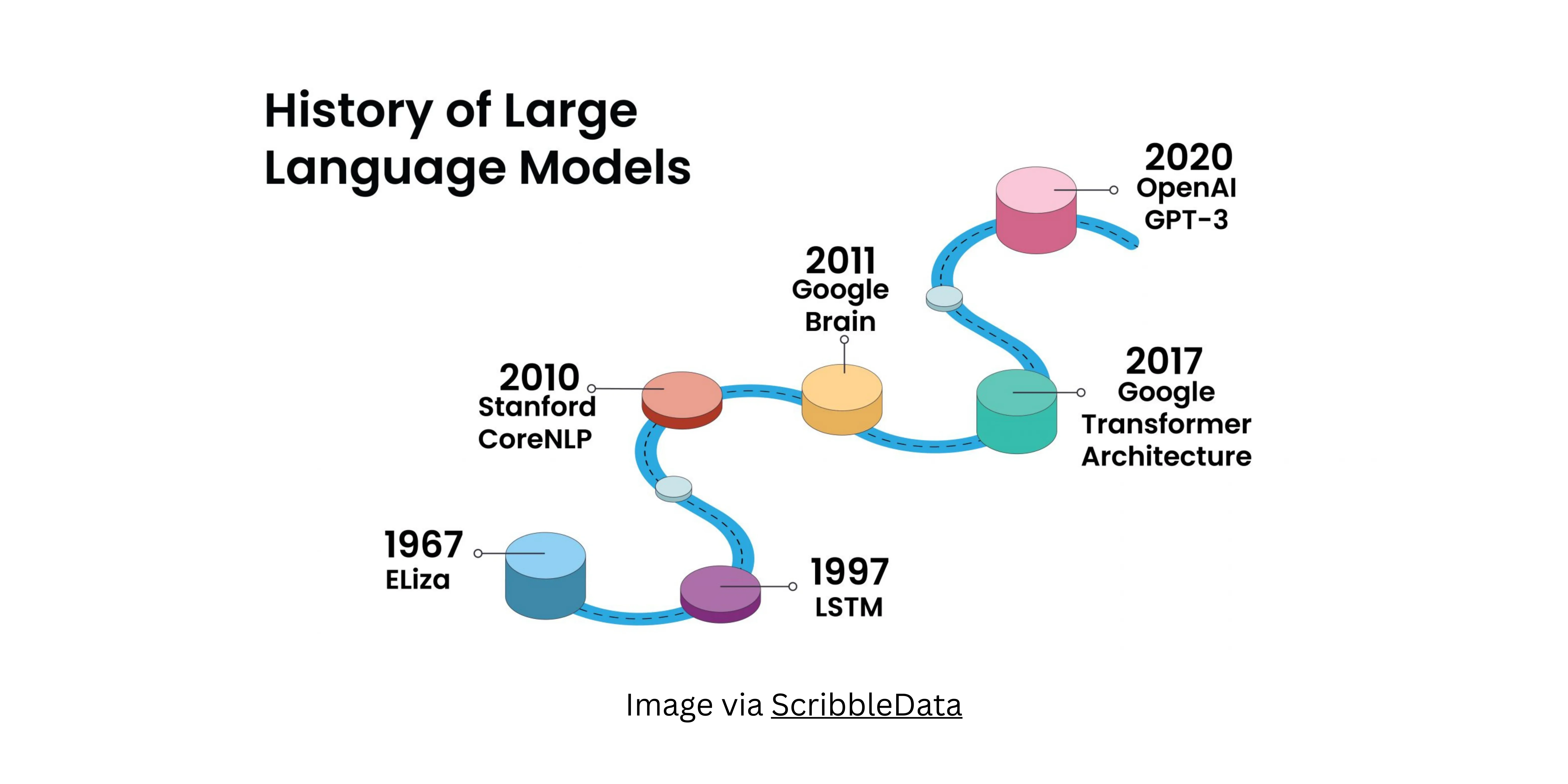
LLM History in Brief
A concise LLM history is described by ScibbleData.io here. Perhaps the most notable event in recent LLM progress occured in 2017, when Google introduced the transformer architecture with a paper titled: “Attention Is All You Need.” This architecture, along with the increased computing power made possible by modern GPUs from Nvidia and others. The photo from Nvidia below shows the expansion of compute that enabled GPT-3 and beyond.
Emerging LLMs are still under development, but as the world is beginning to see with ChatGPT, Bard, and others, they have the potential to revolutionize the way we interact and work. In the future, LLMs may be able to understand and respond to our questions in a way that is largely indistinguishable from a human. LLMs may also begin to solve problems in ways human beings simply cannot.
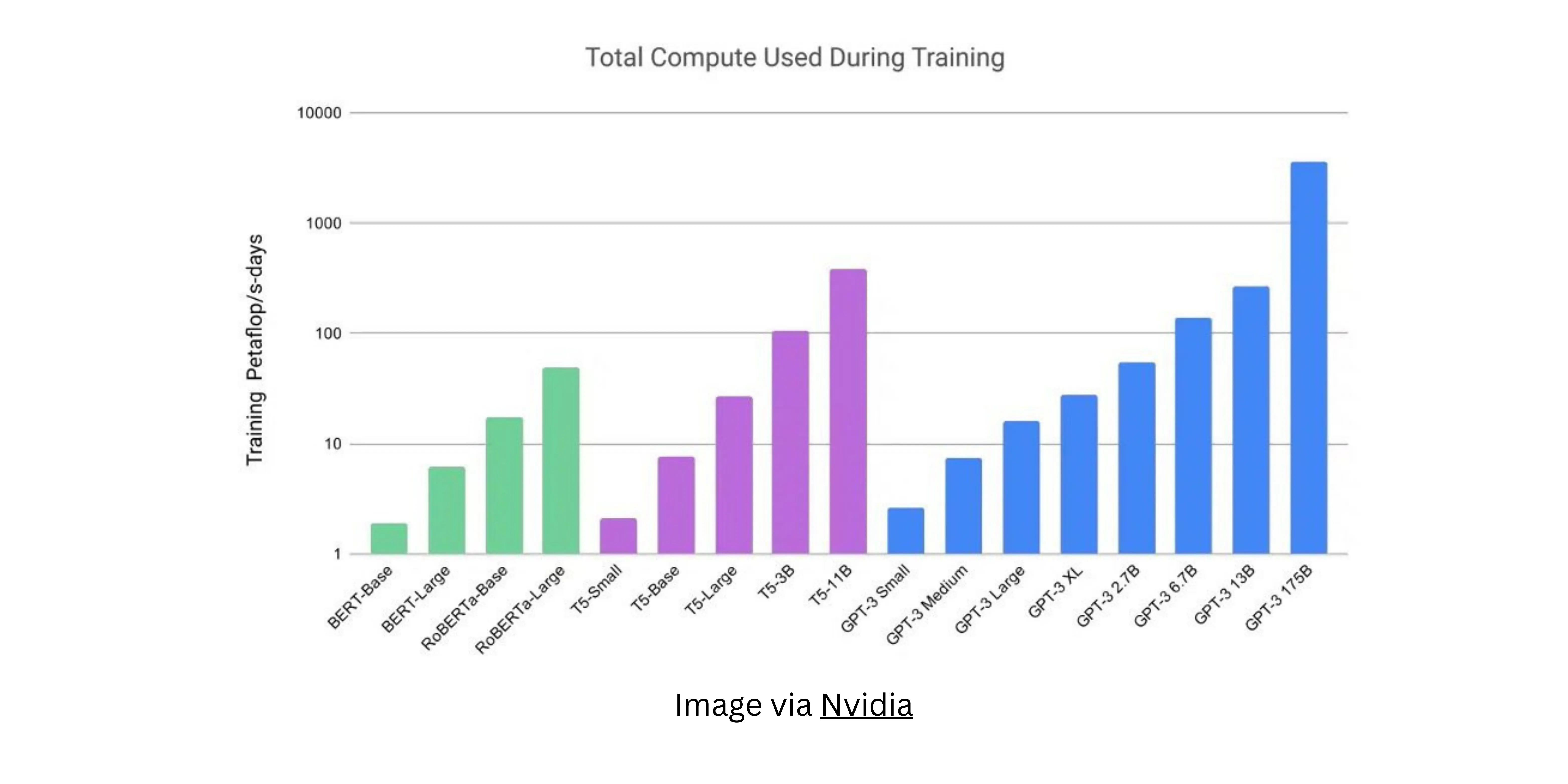
What's Next for LLMs?
Given the current progress of LLMs and their recent exponential growth, it is reasonable to believe there are at least 4 critical areas LLMs will improve in the next few years. 4 identified areas are as follows:
- Speed: Agents do more in less time
- Personalization: Agents will automatically generate custom responses for each user, based on their specific needs
- Context: Agents become better at understanding context
- Multimodal Input: Almost all LLMs are currently good at text, some are good at speech, the best ones will become great at recieving info from essential any form (text, voice, photos, videos, etc.) and generating excellent responses.
Conclusion
ScholarAI's journey with ChatGPT reflects a commitment to enhancing the accessibility, trustworthiness and reliability of information in the academic world. As we continue to serve millions of requests and refine our features, the future of LLMs in research looks promising, characterized by increased speed, personalized context understanding, and multimodal input capabilities.
With ScholarAI, the landscape of research is not just evolving; it is transforming, promising a more interconnected and intelligent approach to knowledge discovery.
If you're ready to take the next step in elevating your research journey, join our journey toward reliable and accurate AI with over 200M+ scholarly articles accessible - sign up for ScholarAI here.